Data Analytics Success Stories
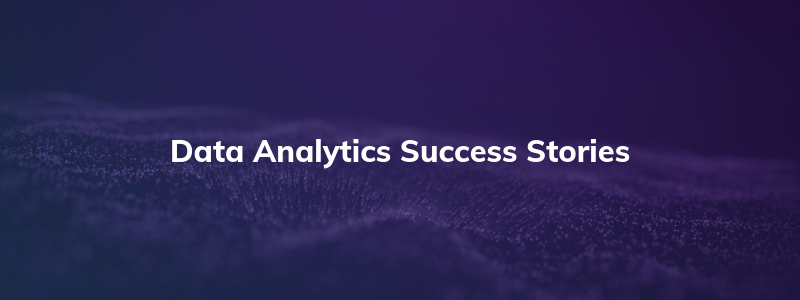
Organizations are able to generate more data than ever before with the help of artificial intelligence (AI) and machine learning (ML). Interestingly, this inflow of information is the reason why the data scientist job title was crowned the “most promising job” of 2019 – which has held the number one spot on Glassdoor’s Best Jobs in America list for the last four consecutive years.
Enterprises are generating enormous quantities of data from a variety of diverse sources – whether it’s from the enterprise systems themselves, or from social media or other online sources, from smartphones and other client/edge computing devices, or from sensors and instruments encompassing the Internet of Things. This data is tremendously valuable to the companies that have the tools in place to capitalize it. And the overall toolbox for these tools is called data analytics.
Specifically, data analytics is a broad term that refers to the use of various techniques that find meaningful patterns in data. It is a method by which data is converted into insight and foresight. Data analytics tools allow organizations to define what transpired in the past, obtain insights about the present-day, and — with some systems — compose forecasts about what’s in store for the future.
The use of data analytics will only continue to grow in the future, as it is a necessary tool for organizations to use when it comes to making big business decisions. Gartner predicts that by 2022, 90 percent of corporate strategies will overtly mention information as a critical enterprise asset and analytics as an essential competency.
Three Data Analytics Success Stories:
· Shell Learns to Anticipate Machine Failure
Very few sectors generate more data than the energy industry. But for many years, the oil giant did not know where parts were in its various facilities around the world. It also didn’t know when to restock and when maintenance issues were occurring – until parts began to fail. Machine downtime can cost industrial companies millions of dollars a day, so Shell decided to harvest data to head off these issues.
Shell constructed an analytics platform based on software from several vendors to run predictive models to anticipate when more than 3,000 different oil drilling machine parts might fail, according to Daniel Jeavons, general manager of Shell’s data science center of excellence. One of those tools, Databricks, captures streaming data via Apache Spark. Shell uses this tool to better plan when to purchase machine parts, how long to keep them, and where to place inventory items. The tool, hosted in Microsoft Azure’s cloud, has helped Shell reduced inventory analysis from over 48 hours to less than 45 minutes, shaving millions of dollars a year off the cost of moving and reallocating inventory.
· TD Bank Reimagines its Enterprise Data Platform
TD Bank’s data analytics team spent several years updating its data infrastructure for current and future needs – creating an enterprise Hadoop data lake. According to Joe DosSantos, TD Bank’s senior vice president of enterprise information, the Cloudera-based lake is used to cultivate insights about customers, which includes tracking attrition rates to targeting just the right products. One of TD Bank’s core focuses is giving business analysts the ability to pull data from the data lake and make it useable and actionable – without requiring data scientists to help them throughout the entire process.
· Merck Creates a Data Warehousing System
Merck, a global healthcare company, wanted to use data collected in ERP and core systems for manufacturing execution and inventory control to gain additional business insights. But since Merck’s engineers spent 60 percent to 80 percent of their effort finding, accessing and ingesting data for each project, the business objective was being unfulfilled. "We were not viewing data as a viable, permanent and valuable asset," says Michelle D'Alessandro, CIO of manufacturing IT at Merck. "We wanted to establish a culture where we spent far less time moving and reporting the data and far more time using the data for meaningful business outcomes."
Merck created MANTIS (Manufacturing and Analytics Intelligence), a data warehousing system comprising in-memory databases and open source tools. MANTIS can crunch data housed in structured and unstructured systems, including text, video and social media. In addition, the system was designed to allow non-technical business analysts to easily see data in visualization software. But on the other hand, data scientists were able to access information through sophisticated simulation and modeling tools. Overall, MANTIS helped decrease the time and cost of the company's overall portfolio of IT analytics projects by roughly 45 percent. The tangible business outcomes included a 30 percent reduction in average lead time, and a 50 percent reduction in average inventory carrying costs.