Develop "Agile" data lakes – or risk a data swamp
Add bookmark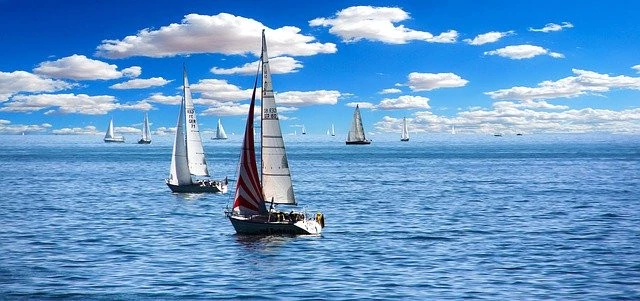
It is estimated – from various sources – that the world will have generated around 40 trillion gigabytes of data in the course of 2020. This shows the unprecedented rate of technology and internet usage. I won’t be surprised if we surpass this estimate.
Loads of data means loads of problems to deal with, as someone needs to manage data, generate insights and store it appropriately.
Data arrive in all formats – structured and unstructured; and from various sources, including Internet-of-Things devices, social-media sites, sales systems, and internal-collaboration systems.
A few decades ago, data warehouses were the key solution. They were meant to store structured data and have special security features. They were also well integrated with various ERP systems. However, data warehouses are not cut out to handle large volume of data and don't have the ability to deliver with speed. Executives were under pressure to massively upgrade their infrastructure and focus on providing insights from data.
Thus was born the concept of ... “data lakes!”
Data lakes can store data in all formats. Their biggest strength is the ability to store unstructured data from any source and in any format including original and raw formats. In their truest form, data lakes had the following advantages:
- Analytics Agility – Data lakes turned out to be a gold mine for data scientists as they can access data quickly from a single source in native form or processed form and generate meaningful insights.
- Reduced Costs – With increasing volumes of data, data lakes offer a cheaper way to store and ingest data on one platform.
However, data lakes come with their own sets of challenges:
Problems started arising when everyone wanted to create a data lake for themselves. It resulted in siloes and multiple data lakes within the same organization. Different functions within the organization have different business rules, however, so the source and complexity of data also varies. The result is a slowing down of data lakes programs as firms are waiting to create an enterprise-wide consistent approach.
The worse situation is when organizations end up with a massive, inconsistent data repository, with no clear rules. In their excitement, firms start dumping all types of data into data lakes, without any clear strategy.
The importance of an Agile approach in building data lakes
Companies need to apply an agile approach to design and roll out data lakes. It’s always better to adopt a flexible approach and build your data lake step-by-step (pilot by pilot and then scale). Firms can build a data lake with certain functional requirements and they can keep adding or changing business rules, or adopt new regulations or business requirements subsequently. An Agile approach results in quick delivery and helps firms realize benefits earlier. IT, along with business leaders, can prioritize business/functional use cases requirements that needs to be implemented first.
Quite often the project faces challenges in terms of unclear requirements, poor data quality from source, choice of tools and technology, etc. A smart approach (Agile) is to develop a data lake incrementally and adopt selected use cases – basically, start with a pilot and gradually scale.
The agile approach also helps in identifying challenges at earlier an stage. If there are issues with respect to performance or quality they will be caught earlier and can be fixed. This also allows you to incorporate business feedback – and data scientists get access to data fairly quickly to start building insights; their feedback further strengthens the design of data lakes.
Successful organizations have learned over a period of time that data lakes can quickly turn into ugly programs if not planned and executed properly. Firms will do well if they do not take the approach of creating massive data lakes with all functional sources in one go.
Instead, they will be better off with an agile approach of creating piece-by-piece, with robust and flexible designs that can be scaled as required.