“Averages Are Lazy” – Experimenting with Analysis & Visualization in Measuring Shared Services Customer Experience
Add bookmark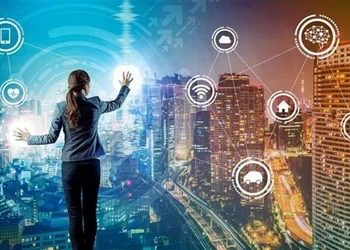
Following our experiments that gave rise to “frustration-free” as our standard anchor question, we began looking for good ways to analyze questions with 5-point Likert scale responses.
Another of my favorite cultural norms at Amazon (most of them outgrowths of particularly pithy Jeff Bezos quotes), is the observation that “averages are lazy.”
We immediately recognized that aphorism’s relevance to our measurement challenges – we’d already seen that relying on Mean scores alone would often yield scant insight (for example, some processes manifest elements of Reversion to the Mean, becoming so stable over time that it becomes difficult to identify meaningful shifts in experience).
We also saw the challenge of attempting to compare services using only Means, a technique that frequently masks too much underlying context. To illustrate, consider the case of 4 hypothetical services, with identical Mean experience scores on a 5-point scale:
Service: |
Service W |
Service X |
Service Y |
Service Z |
Mean: |
3.05 |
3.05 |
3.05 |
3.05 |
Not much you can do with that. Middling scores that coincidentally match. So you ask your friendly neighborhood analyst for help and they take you one click deeper:
Service: |
Service A |
Service B |
Service C |
Service D |
Mean: |
3.05 |
3.05 |
3.05 |
3.05 |
Standard Deviation: |
0.99 |
1.21 |
1.41 |
1.65 |
This raises a little flag – larger standard deviations suggest wider variation in experience – implying that some processes may be out of control (in the Statistical Process Control sense). The flag gives you reason to analyze further, but doesn’t necessarily ease the tracking of ongoing service health.
So you again phone a friend, and the analyst suggests a simple visualization to shed a little more light:
Service W illustrates your stereotypical Normal Distribution, Service X a Bimodal Distribution, Service Y an uninspiringly undifferentiated result, and Service Z an even more extreme, concave version of Service X’s Bimodal Distribution, but with far less Central Tendency.
Four very different underlying service experiences, becoming clearer as we iteratively dive deeper. Not a bad start to analyzing services. But ever hungry for deeper insight, you want more.
*********
Because of the large number of experiences we measure globally, and because leaders’ time and mindshare are often overtaxed, when looking for ways to concisely represent survey analyses, we again turned to a practice historically used in Amazon’s Customer Service organization – converting 5-point Likert results into Positive Response Rate (PRR = the proportion of respondents choosing the top two options) and Negative Response Rate (NRR = the proportion choosing the bottom two).
We liked the approach because it consolidated what marketers would call your “Love Group” and your “Hate Group” into two obvious segments, while not giving you “credit” for neutral responses in either segment. It also allowed leaders to more quickly absorb a service’s health. PRR and NRR can be stark reminders of what’s working and what’s not, by not hiding behind a Mean nor by requiring absorption of a dashboard swimming in bar graphs.
“There are many advantages to a customer-centric approach, but here’s the big one: customers are always beautifully, wonderfully dissatisfied, even when they report being happy and business is great. Even when they don’t yet know it, customers want something better, and your desire to delight customers will drive you to invent on their behalf.” –Jeff Bezos, 2016 Shareholder Letter
As our services became more comfortable with PRR/NRR-oriented goals, we looked for ways to more effectively represent how a service’s health shifts over time, or how services perform relative to one another. To that end, we’re beginning to leverage the Ternary Plot.
I was first exposed to the technique during my early professional days helping manage a family greenhouse/nursery business. I was trained as a certified Master Gardener, and during the classes spent a riveting evening (irony mine) learning to identify various soil textures and their impact on plant health. But one tool engaged my nascent geek – using a Ternary Plot to identify soil type by its underlying components. I confess I came to enjoy testing soil when I found I could plot my findings on a Ternary diagram, then help my customers identify concrete ways (entendre also mine) to improve their gardens. Eventually I realized such a tool could be useful in other contexts, but didn’t land on any good use-cases until I came to Amazon, with measuring service health being the most recent.
*********
Using a Ternary Plot is simple enough: you identify the percentages of any 3 variables (in our case PRR, NRR, and Neutral) and find their intersection on the triangular plotting field. We use it as another way to categorize service health. As mentioned, we’ve begun using the tool in two ways:
1) To compare service health across services:
2) To track the change in a single service’s customer experience over time:
While nobody loves a label, categorizing services in this way allows quick comparisons among disparate services, and can help suggest where to conduct internal benchmarking – another service that has “been there” before can share practices to help bridge customer experience gaps based on where your service is currently performing.
Using some of the approaches above help us avoid the statistician’s quip regarding the perils of Mean: “With your head in the oven and your feet in the freezer, on average you’re fine.”
For more on how we pursue deeper quantitative and qualitative insights, then take action to improve customer experience, watch for additional articles here soon.
[eventpdf]