How to Determine the Right Document Reading Solution for Your Operation
Add bookmark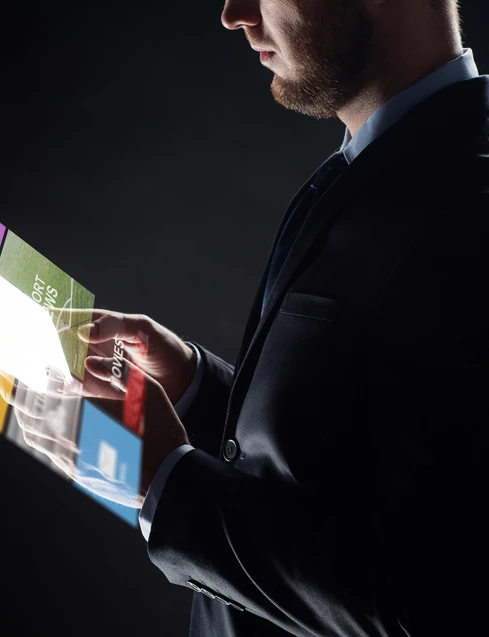
SSON speaks with analyst NelsonHall about their new SmartLabTest evaluation of some of the leading document cognition platforms
In most modern enterprises, business services leaders have recognized that real value derives from seamless processing – the ability to manage an end-to-end process workflow without encountering friction that results in exceptions, bottlenecks or workarounds. Modern process automation solutions have stepped into the breach to facilitate touchless workflows and have, indeed, driven significant performance improvements.
One of the ongoing challenges, however, has been the (in)ability to access, read and act on data, which takes multiple forms within the modern enterprise. Seamless processing relies on digital inputs, and yet most enterprise data is not yet digital, despite the trend moving in this direction.
One of the ongoing challenges has been the (in)ability to access, read and act on data.
These non-digital inputs present a significant hurdle to performance. Document reading, therefore, is the first step in end-to-end automation. Said differently: The success of process automation depends to a large extent on the ability to read and leverage relevant data along the way.
Many of the leading automation vendors have recognized this limiting factor and have been busy developing what are broadly termed “document cognition” solutions. These are able to “read” non-digital data, which is then assimilated into ongoing process activity. Many of these solutions claim to read and extract various data structures with a high degree of success.
The challenge, however, is that there are few apples-to-apples comparisons of these capabilities. Indeed, plenty of analyst assessments simply reflect vendors’ marketing messages without being subjected to rigid third-party testing.
There are few apples-to-apples comparisons of these capabilities... buyers are not being presented with reliable comparisons when it comes to solutions’ ability to read various data forms successfully.
“One of the challenges that we find end-users are confronted with is that they are promised significant reliability in reading non-digital content, which inevitably is not borne out in practice,” explains Mike Smart, Intelligent Automation Platform Research Analyst at NelsonHall. “In particular, buyers are not being presented with reliable comparisons when it comes to solutions’ ability to read various data forms successfully.”
To plug this gap, NelsonHall has just published its SmartLabTest evaluation of five of the leading document cognition platforms. The objective, Mike says, is to provide comparative data on how each platform performs across structured, semi-structured, and unstructured data.
“The key differentiator in this evaluation is that we used the same 10 test documents across each of these platforms, and the same set of training documents,” says Mike. “We analyzed the ability to find the relevant data, the accuracy of this reading, and how many exceptions were initiated as a result. What we believe this evaluation does is highlight the best fit of a given solution, depending on the data to be processed.”
On a more granular level, these documents included structured data (American mortgage documents and ACCORD filings), semi-structured data (invoices, purchase orders and SEC filings), and unstructured data (resumes) formats. All documents other than SEC filings were presented in image form.
“What we found was an enormous variation in different platforms’ abilities to read data,” explains Mike Smart. “Some excelled at reading unstructured data, others at structured. There was also significant variation in ease of use.”
Enormous variation in different platforms’ abilities to read data
The SmartLabTest measures three key criteria. The first is a measure of the percentage of fields recognized (for example, in an invoice that might mean the ability to find the address); the second relates to the accuracy of the data extracted from the fields; and the third measures the percentage of fields that require no intervention (i.e., straight through processing).
The real take away, explains Mike, is that end-users need to be aware of the type of document they are processing through automation in order to select the correct cognition tool. “Most solutions read structured data much better than they do unstructured data, which comes as no surprise, as reading can be based on a template,” he explains. The findings regarding unstructured data are perhaps more insightful. Here, solutions rely on NLP-type capabilities or fractal analysis to identify data on a page and ingest it.
“What we found, perhaps unsurprisingly, is that platforms that performed well on one type of data structure didn’t necessarily perform well across the other two,” says Mike. “What this means is that enterprises need to pick their platform carefully, based on the type of documents they want to process. And, obviously, there is a cost trade-off that guides these decisions.”
“The right decision will depend on the type of document priority. If this is processing resumes for HR, then a platform that successfully delivers on unstructured data will be the right choice,” adds Mike. “However, another point to make is that it’s a lot easier to improve the reading of structured data through training sets and Machine Learning. For unstructured data, on the other hand, it’s a lot harder – and slower – to drive these kinds of improvements.”
The findings of NelsonHall’s SmartLabTest evaluation are released this week through a comprehensive report, and provide all the metrics for each platform and document type, regarding their ability to find a field and extract the correct data.
For more about NelsonHall’s SmartLabTest evaluation, and to access the report see here.