A Prelude to Intelligent Automation: Preparation is Key
Add bookmark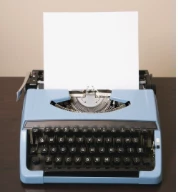
In the digital age the canvas of Intelligent Automation (IA) comprising of Artificial Intelligence (AI), Machine Learning, Deep Learning, Predictive Analytics, Natural Language Processing etc. is expanding rapidly. Many organizations including Shared Services, and BPOs are investing in IA to ensure they are not left behind in the digital race. Futurists are predicting that in the coming times the tribe of digital workers will witness an exponential growth and touch every aspect of human life.
While the interest in embracing IA is heartening, the purpose of this article is to dwell upon some of nuances to be considered before implementing IA solutions.
To start with, the environment is a key consideration for enterprises keen to move ahead with IA. Organizations need to skim through their environment and then articulate the business case for IA. In this context, business verticals or sectors such as finance, insurance etc. have a different environment when compared to media, telecom, healthcare, manufacturing etc. Finance deals with money and is highly regulated when compared to manufacturing, which is largely product or output driven. As the environments are spread across multiple geographies the dynamics of the market and of customers need to be factored in while considering IA. Also, an evaluation of whether IA can be transposed blindly in all environments is required. This will trigger insights into scalability, which is currently a challenge for many organizations who are early movers in the IA space.
Systems and tools inter-linked to the environment constitute another dimension for IA. For example verticals like finance operate with multiple legacy systems which further interface with an Enterprise Resource Planning (ERP) platform and form a complex inter-play of platform and systems/tools. In the present times many organizations are comfortable to invest in upgrade of their legacy systems/tools which may blunt the business case for IA. It would be worthwhile to evaluate whether pure-play workflows and ERPs are a better proposition than replacing or integrating them with IA solutions. Considering that IA is only 5-6 years old there are many emerging vendors and product companies that are bringing newer technological features or products to the market. For example, there is a new AI virtual assistant launched in the market which can now interface with an ERP and automate the ERP processes.
Other pertinent pointers would be the quantum and quality of data stored in the legacy systems/tools and how much risk is involved while adopting an IA solution. A recent study released by McKinsey says that one of the barriers limiting the application of AI technology pertains to data. Datasets play a pivotal role in the adoption of IA solutions and organizations need to be cognizant about the sanctity of the data. The integration of data derived from multiple channels of an enterprise needs careful attention to avoid any defect in the proposed IA solution. Understanding, labelling and segmenting the data are key inputs to determine the success rate of an IA solution. However, the enterprises that are early entrants in this space seem to be acknowledging this lately after experiencing setbacks in the models.
The current sentiment in the industry is to automate and digitize all processes. Here, one needs to evaluate the right fit of processes including the length and breadth of IA. Today, many large enterprises are wired in legacy processes and tools having multiple hand-offs and seem to be comfortable with the status quo. Given the large size of these enterprises and the headcount there may be headwinds in terms of quickly switching over or adopting an IA solution. For IA to prove itself it is imperative to have a better understanding of processes, business rules and regulations.
As per an IDC survey, only about 30 percent of companies reported a 90 percent success rate for IA projects.
Industry reports and case studies are today proclaiming the replacement of people or human workers by machines. However many unanswered questions pertaining to labour regulations, employee benefits, severance, workplace policies etc. remain, which need to be dealt in a sensitive manner by the enterprises and the governments. A shortage of new talent and re-skilling of existing talent needs to be a priority. Redundancy of roles at all levels in an enterprise will force enterprises to revisit and redraw their target operating models. A divide could emerge between digitally equipped and non-digitally equipped employees in the near future within the enterprise, which could lead to wider ramifications.
In terms of risk, there needs to be absolute clarity on understanding and satisfying the compliance and regulatory parameters. Of late there are questions being posed on algorithmic biases, governance, violation of privacy or individual rights, data management, social norms pertaining to discrimination and inequality, ethics and morality. For example in the field of Artificial Intelligence there is growing evidence of concerns around machine errors and how technology is impairing the cognitive skill of humans. At the moment, IA practitioners are yet to offer convincing solutions and safeguards to mitigate these inherent risks.
With each passing day the interest in IA continues to soar across the globe. Enterprises and corporates are incorporating IA as the fulcrum of their strategies. However they need to dig deeper in the prelude to IA to avoid any shadows on the transformative experience being promised to customers and end users.
[inlinead]